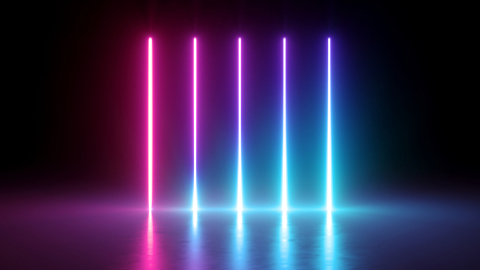
Researchers at UCLA have used Artificial Intelligence (AI) to turn two-dimensional (2D) images into stacks of virtual three-dimensional (3D) slices that show the activity inside organisms. Using deep learning techniques, the team devised a technique that extends the capabilities of fluorescence microscopy – a method that allows scientists to precisely label parts of living cells and tissue with dyes that glow under special lighting.
The framework they designed – called “Deep-Z” is able to fix errors or aberrations in images, such as when a sample is tilted or curved. They were also able to demonstrate that the system could take 2D images from one type of microscope and virtually create 3D images of the sample as if they were obtained by another, more advanced microscope. “Deep-Z” was taught using experimental images from a scanning fluorescence microscope. In thousands of training runs, the neural network learned how to take a 2D image and infer accurate 3D slices at different depths within a sample. Further tests using images that were not part of its training produced an excellent match.
Using this technique, specimens are spared from potentially damaging doses of light and it is expected that the system could offer biologists and life science researchers a new tool for 3D imaging that is simpler, faster and much less expensive than current methods.
“This is a very powerful new method that is enabled by deep learning to perform 3D imaging of live specimens, with the least exposure to light, which can be toxic to samples,” said senior author Aydogan Ozcan, professor of electrical and computer engineering at UCLA.