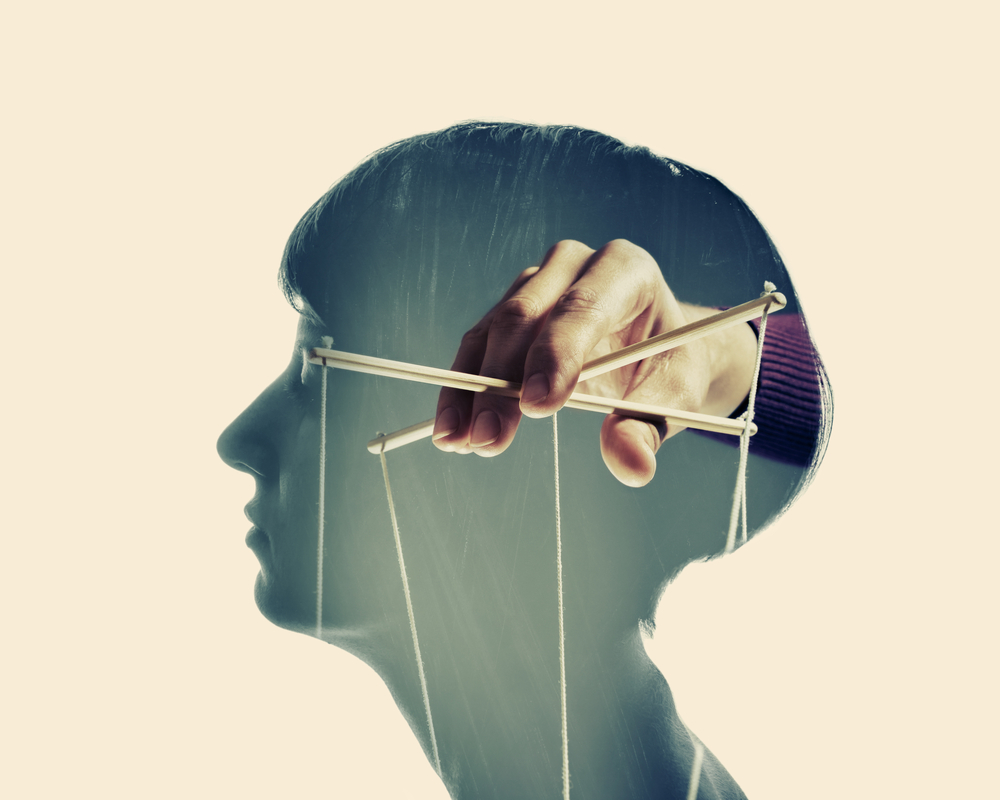
Researchers from the University of Texas at Austin have successfully demonstrated a wheelchair that can be controlled by the users’ mind.
The research team recruited three tetraplegic people for the study. Each participant underwent training sessions three times per week for 2 to 5 months. To control the wheelchair, the participants wore a skullcap that detected their brain activities through electroencephalography (EEG). These signals would then be converted to mechanical commands for the wheelchairs via a brain-machine interface device. The participants practiced controlling the direction of the wheelchair by thinking about moving their body parts.
In the first training session, three participants had similar levels of accuracy in controlling their movements – around 43% to 55%. Over the course of training, the team observed significant improvement in accuracy in participant 1, who reached an accuracy of over 95% by the end of his training. The team also observed an increase in accuracy in participant 3 to 98% halfway through his training before the team updated his device with a new algorithm.
The team concluded that the positive results not only arose from the improved machine learning of the device, but also learning in the brain of the participants. The EEG of participants 1 and 3 showed clear shifts in brainwave patterns as they improved accuracy in mind-controlling the device.
Participant 2 had no significant changes in brain activity patterns throughout the training. His accuracy increased only slightly during the first few sessions, which remained stable for the rest of the training period. The team plans further studies of all the participants’ brain signals to better understand their differences and possible interventions for people struggling with the process in the future.
“We show that mutual learning of both the user and the brain-machine interface algorithm are both important for users to successfully operate such wheelchairs,” says José del R. Millán, the study’s corresponding author. “Our research highlights a potential pathway for improved clinical translation of non-invasive brain-machine interface technology.”