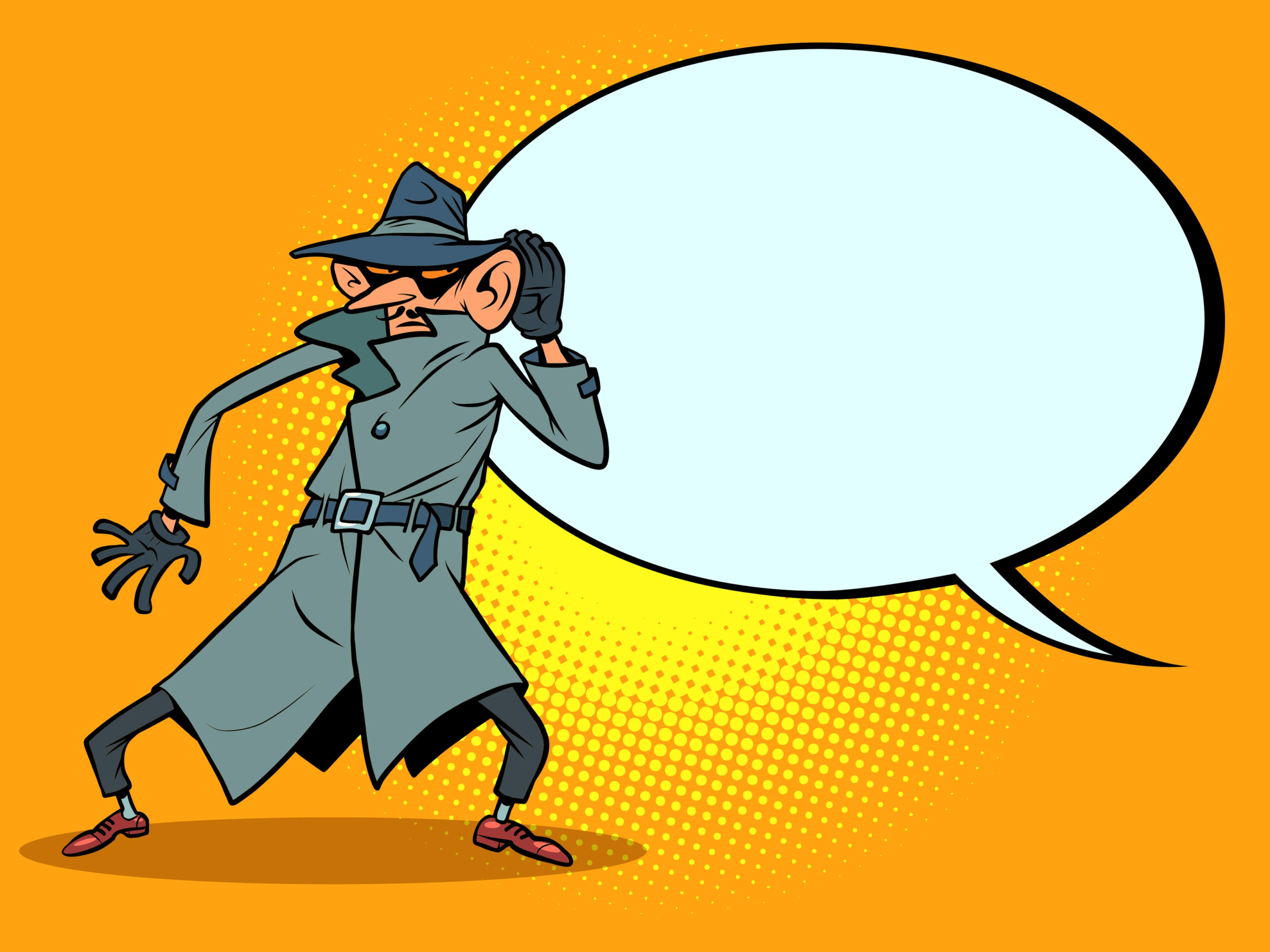
Penn State researchers have identified a significant security concern regarding eavesdropping. By using an off-the-shelf automotive radar sensor and a unique processing approach, they were able to detect the vibrations of a cell phone’s earpiece and decipher what the person on the other side of the call was saying with up to 83% accuracy.
“As technology becomes more reliable and robust over time, the misuse of such sensing technologies by adversaries becomes probable,” Suryoday Basak, one of the researchers said.
The radar operates in the millimeter-wave (mmWave) spectrum – specifically in the bands of 60 to 64 gigahertz and 77 to 81 gigahertz. The team noted that mmWave radars are becoming popular in a number of autonomous driving, remote sensing, and other IoT applications, leading to greater privacy concerns.
The demonstration – called mmSpy – simulated people speaking through the earpiece of a smartphone. Though the brand is irrelevant, the researchers tested their approach on both a Google Pixel 4a and a Samsung Galaxy S20. The phone’s earpiece vibrates from the speech, and that vibration spreads across the body of the phone. The radar is used to sense this vibration and reconstruct what was said by the person on the other side of the line. The system works even when the audio is completely inaudible to both humans and microphones nearby.
The radar sensor data is pre-processed via MATLAB and Python modules, which remove hardware-related and artifact noise from the data. The researchers then feed that to machine learning modules trained to classify speech and reconstruct audio. When the radar senses vibrations from a foot away, the processed speech has an accuracy of 83%, which falls to 43% accuracy at six feet.
Once the speech is reconstructed, the researchers can then filter, enhance, or classify keywords as needed. The team continues to work towards better understanding how to protect against this security vulnerability, and also how it could be beneficial.
“The methodology that we developed can also be used for sensing vibrations in industrial machinery, smart home systems and building-monitoring systems,” Basak said. “Vibration tracking over time can help assess wear and tear – using our approach could help identify when machinery needs maintenance before it would traditionally be obvious, for example.”